Projects
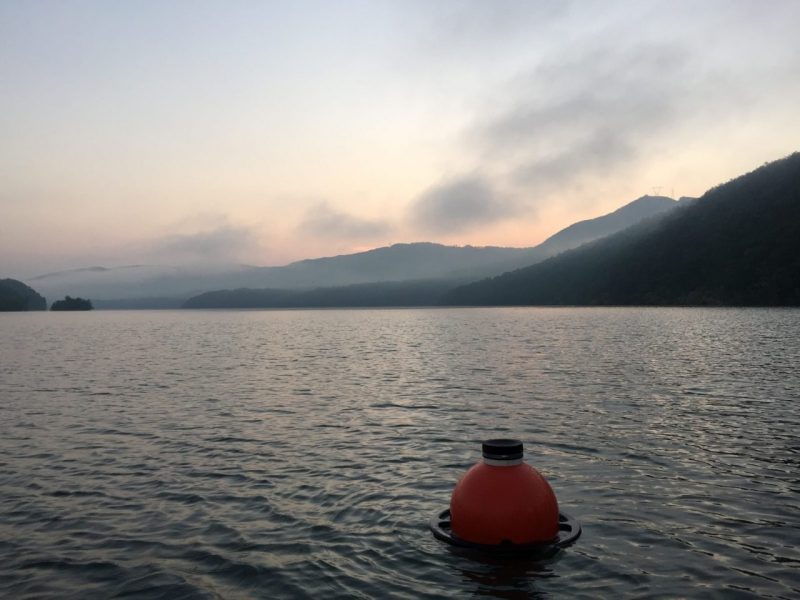
WATER QUALITY
Applying rules of life to forecast emergent behavior of phytoplankton and advance water quality management
Drinking water safety is threatened globally by increasing phytoplankton blooms in lakes and reservoirs, which pose major threats to water quality via harmful toxins, scums, and changes in taste and odor. To improve drinking water management in the face of global change, this project proposes to develop the first automated, real-time lake phytoplankton forecasting system that quantifies uncertainty in water quality predictions. If managers had forecasts of phytoplankton blooms, they could preemptively act to mitigate water quality impairment, such as by adapting water treatment, thereby decreasing costs and improving drinking water safety. The project team plans to integrate cutting-edge lake ecosystem and statistical modeling with new computing capacity to deliver 1 to 35 day-ahead forecasts of phytoplankton blooms to water managers daily for several U.S. lakes. Researchers intend to work with water managers on the forecasting system to generate valuable knowledge about how best to effectively communicate forecasts for improved water resource decision-making.
Funding: National Science Foundation (EF-2318861)
Center personnel: Cayelan Carey, Quinn Thomas, Mary Lofton, Madeline Schreiber, Adrienne Breef-Pilz, Austin Delany, Bobby Grammacy, Ryan Calder
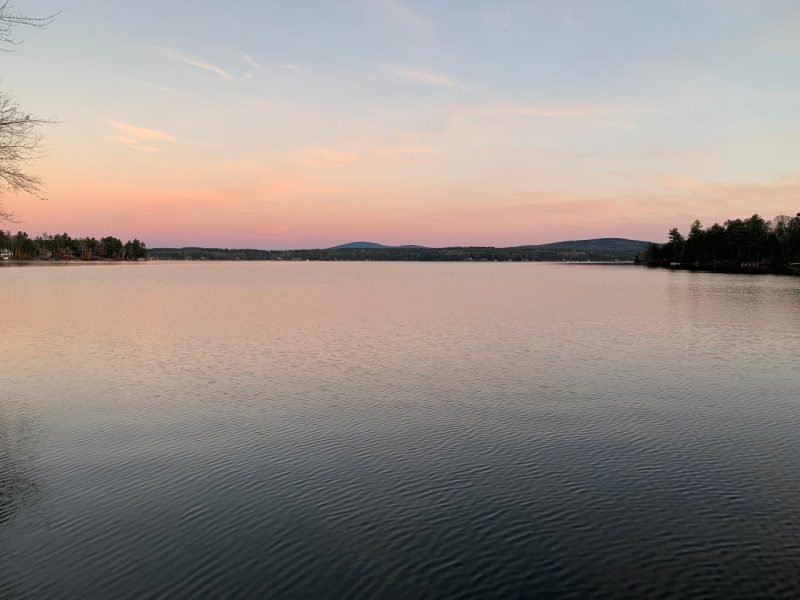
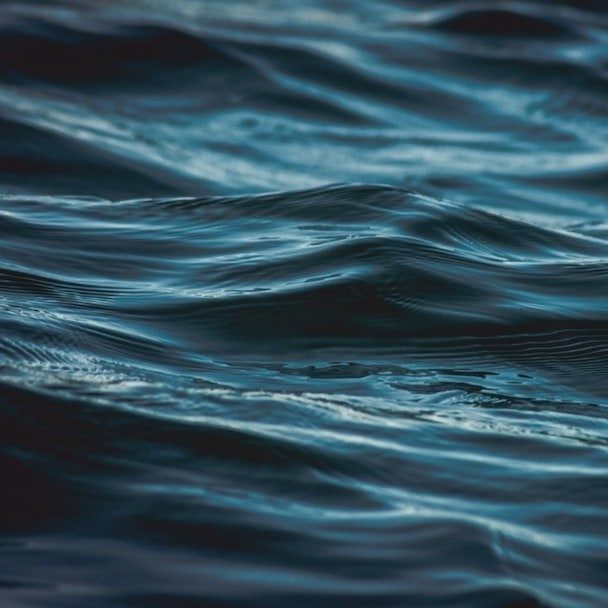
WATER QUALITY
Towards a Global Center for Freshwater Forecasting
Climate change is degrading water quality in lakes and reservoirs around the globe, motivating the urgent need for new approaches to predict future ecosystem and drinking water conditions for management. This Global Centers Track 2 Design project will create the groundwork for developing a global center to provide scientists, managers, and the public in the U.S. and Australia with state-of-the-art, real-time water quality forecasts and decision support tools for lakes and reservoirs. The system will be deployed and tested at four lakes facing a range of climate change threats. Daily forecasts will be generated for a suite of water quality variables and methane emissions, which can inform adaptive monitoring and preemptive management (e.g., divert water from other reservoirs, increase water flows to reduce methane production). Interactive educational materials will be developed and assessed to train managers and community members on freshwater forecasting. A broad community of American and Australian students, academic researchers, government officials, industry representatives, water managers, and the public will be engaged in all center activities, setting the stage for future global engagement via partnerships with multiple international initiatives. The project's overarching goal is to develop the foundation for an international forecasting center that predicts lake and reservoir water quality across the U.S. and Australia to enable climate change adaptation and mitigation.
Funding: National Science Foundation (OISE-2330211)
Center personnel: Cayelan Carey, Quinn Thomas, Freya Olsson
WATER QUALITY
Long-term Research in Environmental Biology
Many ecosystems are exhibiting increased variability as a result of human activities. This environmental variability poses substantial challenges for managers and decision-makers, who can no longer use historical baselines to guide predictions of future ecosystem conditions. Consequently, advancing the capacity to predict the future for a range of physical, chemical, and biological ecosystem variables that influence water quality is paramount for improving resource management. In response to this need, this Long Term Research in Environmental Biology (LTREB) project will support a field monitoring and data-sharing program at two drinking water supply reservoirs. The ecosystem data that will be collected (which will include water temperature, clarity, chemistry, and phytoplankton, among other variables) will be used to generate and evaluate real-time forecasts (predictions of future ecosystem conditions, and the uncertainty associated with them) at daily to annual scales. Forecasting is a powerful approach for quantifying ecological predictability, as it requires using models that represent our best hypotheses about how ecosystems function to predict ecological conditions into the future. Iteratively evaluating these forecasts as new data are collected will reveal which models perform best in different environmental conditions and identify how far into the future different variables can be accurately predicted, from one day to one decade in advance. This project will enable the testing of fundamental hypotheses about the predictability of ecosystems; develop novel workflows for integrating environmental observations into real-time forecasting and data publishing; and broaden the participation of students from underrepresented groups in environmental data science. Moreover, all forecasts will be disseminated to water utilities in real time, enabling their immediate use as decision-making tools for water management.
Funding: National Science Foundation (DEB-2327030)
Center personnel: Cayelan Carey, Quinn Thomas, Madeline Schreiber, Adrienne Breef-Pilz, Austin Delany
Center graduate students:
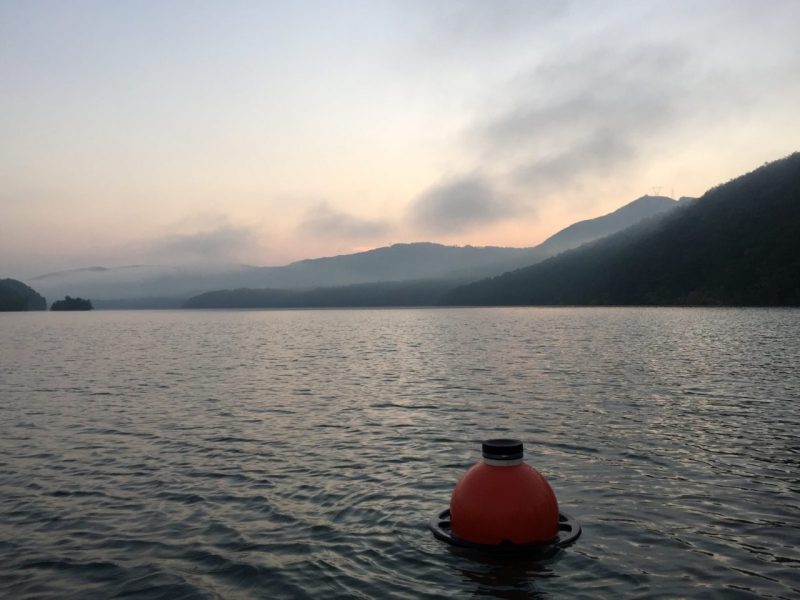
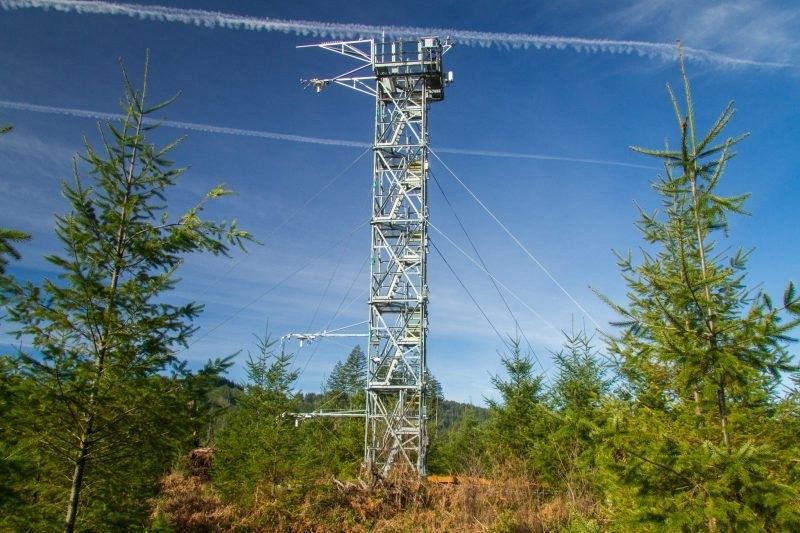
ECOLOGICAL OBSERVATORIES
Ecological Forecasting Initative Research Coordination Network
The 21st century is characterized by major environmental changes that alter the ecosystems society depends on for supplying clean water, storing carbon, and supporting plants and animals. Predicting and forecasting the future of these systems can help resource managers anticipate and respond to these changes. Ecological forecasts also advance scientific understanding by developing and testing models and testing alternative views of how ecological systems operate. To harness the power of forecasting, a community of practice is needed. This community will establish standards for developing and communicating forecasts. It will share best practices for generating forecasts with known uncertainties. The group will create tools and educational materials to enable ecologists to begin forecasting. The Ecological Forecasting Initiative Research Coordination Network award will build this community of practice around forecasting data from the continental-scale National Ecological Observatory Network (NEON). By focusing on data from NEON, the community will be challenged to develop and evaluate forecasts for a diversity of environments that exist across the United States. The award will train hundreds of early career researchers and graduate students in ecological forecasting.
Funding: National Science Foundation (DEB-1926388)
Center personnel: Quinn Thomas, Freya Olsson, Austin Delany, Leah Johnson, Cayelan Carey
EDUCATION
Macrosystems EDDIE: An undergraduate training program in macrosystems science and ecological forecasting
Ecologists are increasingly analyzing big environmental datasets to make forecasts about the future health of ecosystems. However, the data analysis and modeling skills needed to successfully develop ecological forecasts are rarely taught in undergraduate classrooms. To overcome this challenge, this project will expand an existing, successful training program (Macrosystems EDDIE: Environmental Data-Driven Inquiry & Exploration) to teach students fundamental ecological concepts as they create forecasts for lakes and forests across the United States. Through Macrosystems EDDIE, students and instructors will learn how to use models, assess forecast accuracy with observational data, and communicate forecasts to managers and decision-makers. These skills will be embedded in stand-alone teaching modules that will be widely applicable to multiple disciplines and student experience levels. Macrosystems EDDIE provides an innovative new approach for teaching macrosystems ecology and has the potential to advance undergraduate science education across the U.S. By strengthening both students' quantitative skillsets and understanding of macrosystems ecology, this project will help develop a diverse, globally-competitive scientific workforce and enhanced infrastructure for macrosystems research and education.
Funding: National Science Foundation (DEB-1926050)
Center personnel: Cayelan Carey, Mary Lofton, Quinn Thomas
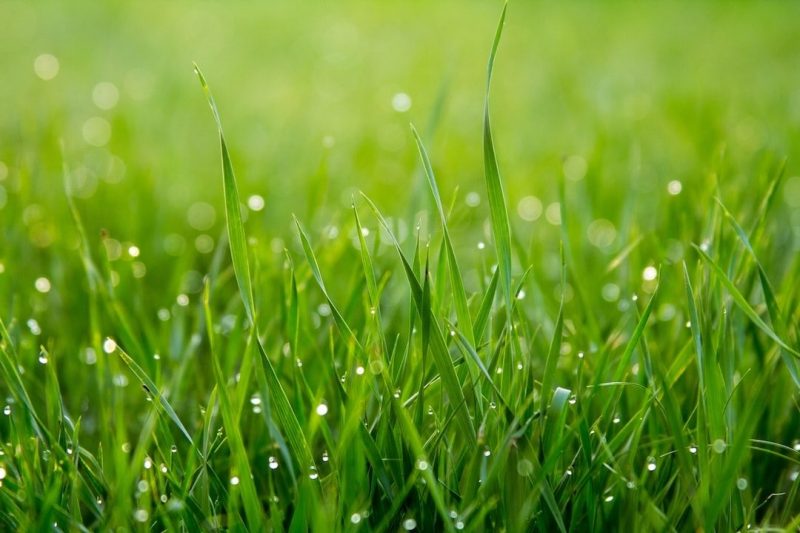
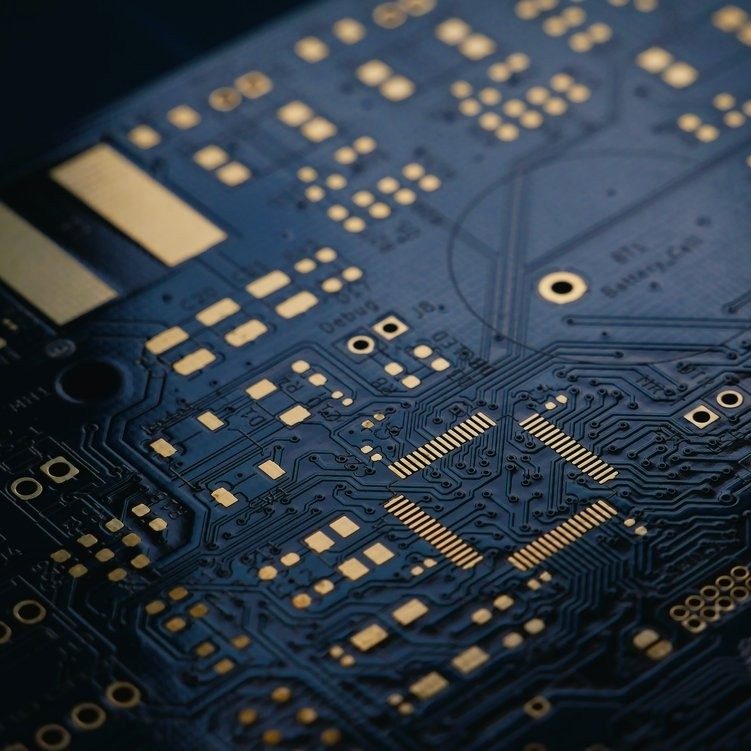
WATER QUALITY
Cyberinfrastructure Enabling End-to-End Workflows for Aquatic Ecosystem Forecasting
Aquatic ecosystems in the United States and around the globe are experiencing increasing variability due to human activities. Provisioning drinking water in the face of rapid change in environmental conditions motivates the need to develop forecasts of future water quality. Near-term water quality forecasts can guide management actions over day to week time scales to mitigate potential disruptions in drinking water and other essential freshwater ecosystem services. To maximize the utility of water quality forecasts for managers and decision-makers, the forecasts must be accessible in near-real time, reliable, and continuously updated with environmental sensor data. However, developing iterative, near-term ecological forecasts requires complex cyber-infrastructure that is widely distributed, from sensors and computers collecting information at freshwater lakes and reservoirs to cloud computing services where forecast models are executed. Consequently, significant software challenges still remain for environmental scientists to easily and effectively deploy forecasting workflows. This project will address this need by designing, implementing, and deploying open-source software (FLARE: Forecasting Lake And Reservoir Ecosystems) that will enable the creation of flexible, scalable, robust, and near-real time iterative ecological forecasts. This software will be tested and widely disseminated to water utilities, drinking water managers, and many other decision-makers. FLARE will greatly advance the capability of the ecological research community to perform near-real time aquatic forecasts.
Funding: National Science Foundation (DBI-1933016)
Center personnel: Cayelan Carey, Quinn Thomas, Mary Lofton, Freya Olsson, Madeline Schreiber, Adrienne Breef-Pilz, Austin Delany
CYBERINFRASTRUCTURE
FaaSr: Enabling Cloud-native Event-driven Function-as-a-Service Computing Workflows in R
This project develops FaaSr, a new software that will facilitate the programming and deployment of scientific computing applications written in the R language in Function-as-a-Service (FaaS) cloud computing infrastructures. The FaaS model of cloud computing supports dynamic, on-demand execution of computing functions in servers that are automatically provisioned and managed, in a way that is both cost-effective and scalable: users do not need to manage cloud servers (including on-demand scaling) nor pay for idle time of unutilized servers. The FaaS model thus has much potential for reducing the complexity and cost of performing scientific computing in cloud infrastructures. To date, however, FaaS platforms have been primarily designed to support Web-based applications, resulting in a major gap between existing FaaS platforms and the scientific community. This gap is particularly evident in the environmental sciences, where R is the focal programming language. This is because: 1) there is no native support for the R language in FaaS platforms, and 2) each FaaS platform has a unique interface to deploy and manage workflows consisting of multiple functions, thereby creating barriers for users to develop and deploy applications on one or more FaaS platforms. This project bridges this gap by developing open-source software to accelerate the adoption of event-driven FaaS workflows for scientific applications. The FaaSr software will be distributed as an easy-to-install R package and will provide simple interfaces to programmers, while supporting multiple open-source and commercial cloud computing infrastructures. The software will support a wide range of scientific computing applications, in particular those that require dynamic event-driven processing (such as forecasting and continuous data quality) in environmental science subfields (including ecology and biodiversity). Ultimately, the project aims to develop scalable, generalizable, and robust workflows that will advance the capacity, practice, and training opportunities for ecological forecasting, an active area of scientific research poised to significantly increase predictive capacity for effective environmental decision-making and management.
Funding: National Science Foundation (OAC-2311124)
Center personnel: Quinn Thomas, Cayelan Carey, Austin Delany


CYBERINFRASTRUCTURE
DeCODER: Democratized Cyberinfrastructure for Open Discovery to Enable Research)
Scientific research in most scientific fields today involves significant amounts of data as well as software to operate on that data. Furthermore, research increasingly requires data from across a number of sources, perhaps even fields. This is a significant challenge for the scientific community, and better solutions are needed in order to enable new discoveries and accelerate the societal impacts from those discoveries. The approach in this project is to follow in the footsteps of the web and it aims to standardize how scientific data is described, allowing for tools addressing the above challenges, such as search engines for scientific data that not only support discoverability but also facilitate the usage of the data.
The DeCODER project will expand and extend the successful EarthCube GeoCODES platform and community to unify data and tool description and re-use across geoscience domains. Building on the NSF CIF21 vision, the EarthCube program was formed to address the technological challenges surrounding data and software within the geosciences. Through extensive interaction with the community this culminated in two key activities around data discovery and reuse. First, the promotion, refinement, and adoption of schema.org to annotate geosciences metadata within distributed repositories so that datasets can be crawled. Second, the promotion and support for the adoption of notebooks to document, share, and reuse software as peer reviewed scholarly objects. A rallying point around these activities was the GeoCODES platform, which allows communities to stand up instances of scientific search engines specific to their domains, while building a community of geoscience data users and developers and, ultimately, reducing the time to science. This project will leverage this effort in the DeCODER platform to enable similar activities and outcomes across scientific communities. This work will continue the endeavor to support the scientific community in the adoption of schema.org and notebooks, facilitating this by providing DeCODER as an open source resource that can be customized by a given scientific community to create lightweight scientific gateways that bring together relevant distributed resources.
Funding: National Science Foundation (OAC-2209866)
Center personnel: Quinn Thomas, Austin Delany